SVM RBF Kernel Parameters With Code Examples
In this post, you will learn about SVM RBF (Radial Basis Function) kernel hyperparameters with the python code example.
Join the DZone community and get the full member experience.
Join For FreeIn this post, you will learn about SVM RBF (Radial Basis Function) kernel hyperparameters with the python code example. The following are the two hyperparameters which you need to know while training a machine learning model with SVM and RBF kernel:
- Gamma
- C (also called regularization parameter)
Knowing the concepts on SVM parameters such as Gamma and C used with RBF kernel will enable you to select the appropriate values of Gamma and C and train the most optimal model using the SVM algorithm. Let's understand why we should use kernel functions such as RBF.
Why Use RBF Kernel?
When the data set is linearly inseparable or in other words, the data set is non-linear, it is recommended to use kernel functions such as RBF. For a linearly separable dataset (linear dataset) one could use linear kernel function (kernel="linear"). Getting a good understanding of when to use kernel functions will help train the most optimal model using the SVM algorithm. We will use Sklearn Breast Cancer data set to understand SVM RBF kernel concepts in this post. The scatter plot given below represents the fact that the dataset is linearly inseparable and it may be a good idea to apply the kernel method for training the model.
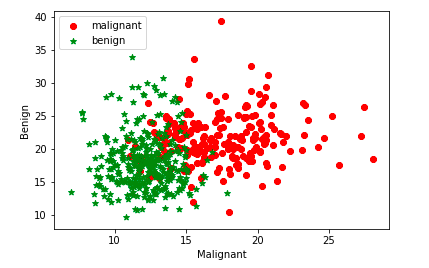
The above plot is created using first two attributes of the sklearn breast cancer dataset as shown in the code sample below:
import pandas as pd
import matplotlib.pyplot as plt
from sklearn import datasets
# Load the breast cancer dataset
#
bc = datasets.load_breast_cancer()
df = pd.DataFrame(data=bc.data)
df["label"] = bc.target
# Scatter plot shown in fig 1
#
plt.scatter(df[0][df["label"] == 0], df[1][df["label"] == 0],
color='red', marker='o', label='malignant')
plt.scatter(df[0][df["label"] == 1], df[1][df["label"] == 1],
color='green', marker='*', label='benign')
plt.xlabel('Malignant')
plt.ylabel('Benign')
plt.legend(loc='upper left')
plt.show()
Given that the dataset is non-linear, it is recommended to use kernel method and hence kernel function such as RBF.
SVM RBF Kernel Function and Parameters
When using the SVM RBF kernel to train the model, one can use the following parameters:
Kernel Parameter - Gamma Values
The gamma parameter defines how far the influence of a single training example reaches, with low values meaning 'far' and high values meaning 'close'. The lower values of gamma result in models with lower accuracy and the same as the higher values of gamma. It is the intermediate values of gamma which gives a model with good decision boundaries. The same is shown in the plots given in fig 2.
The plots below represent decision boundaries for different values of gamma with the value of C set as 0.1 for illustration purposes. Note that as the Gamma value increases, the decision boundaries classify the points correctly. However, after a certain point (Gamma = 1.0 and onwards in the diagram below), the model accuracy decreases. It can thus be understood that the selection of appropriate values of Gamma is important. Here is the code which is used.
xxxxxxxxxx
svm = SVC(kernel='rbf', random_state=1, gamma=0.008, C=0.1)
svm.fit(X_train_std, y_train)
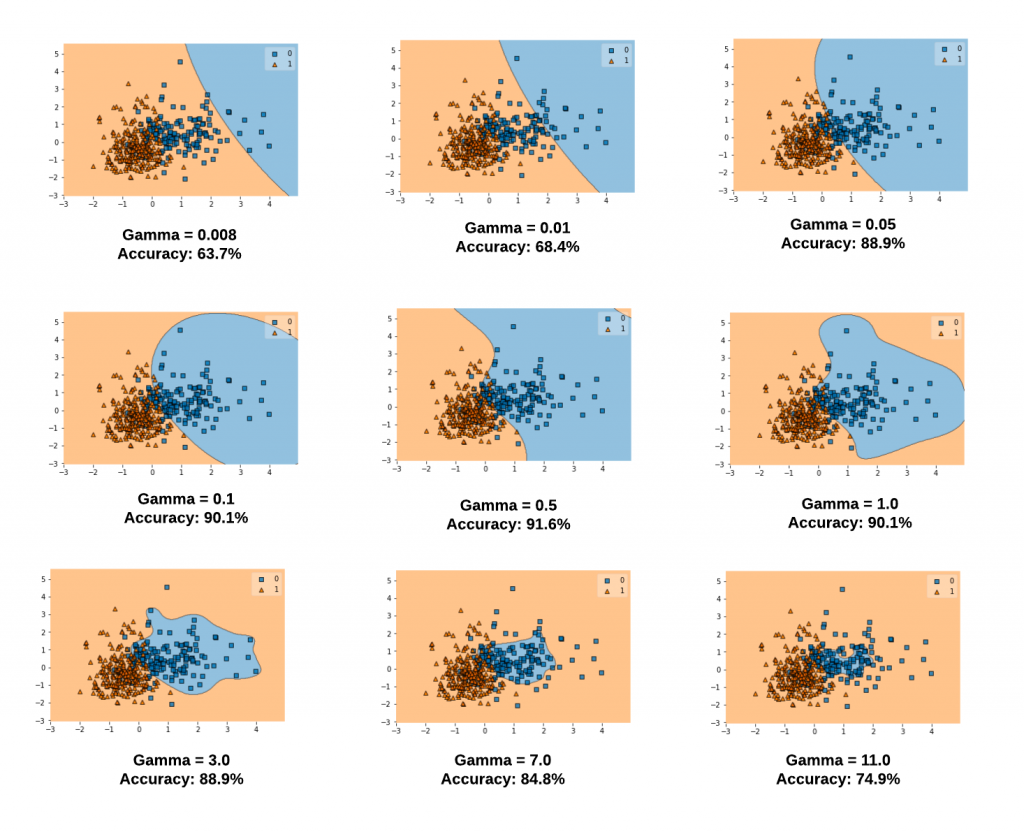
Note some of the following in the above plots:
- When gamma is very small (0.008 or 0.01), the model is too constrained and cannot capture the complexity or "shape" of the data. The region of influence of any selected support vector would include the whole training set. The resulting model will behave similarly to a linear model with a set of hyperplanes that separate the centers of a high density of any pair of two classes. Compare with the diagram in the next section where the decision boundaries for a model trained with a linear kernel is shown.
- For intermediate values of gamma (0.05, 0.1, 0.5), it can see on the second plot that good models can be found.
- For larger values of gamma (3.0, 7.0, 11.0) in the above plot, the radius of the area of influence of the support vectors only includes the support vector itself and no amount of regularization with C will be able to prevent overfitting.
Kernel Parameter - C Values
Simply speaking, the C parameter is a regularization parameter used to set the tolerance of the model to allow the misclassification of data points in order to achieve lower generalization error. Higher the value of C, lesser is the tolerance and what is trained is a maximum-margin classifier. Smaller the value of C, larger is the tolerance of misclassification and what gets trained is a soft-margin classifier that generalizes better than maximum-margin classifier. The C value controls the penalty of misclassification. A large value of C would result in a higher penalty for misclassification and a smaller value of C will result in a smaller penalty of misclassification. With a larger value of C, a smaller margin will be accepted if the decision function is better at classifying all training points correctly. The model may overfit with the training dataset. A lower C will encourage a larger margin, therefore a simpler decision function, at the cost of training accuracy.
The diagram below represents the decision boundary with different values of C for a model trained with a linear kernel and Sklearn Breast Cancer dataset. Take note of the decision boundary for different values of C. Note that as the value of C increases, the model accuracy increases. This goes in line what we learnt earlier that a smaller value of C allows for greater misclassification and hence the model accuracy will be lower. However, after a certain point (C=1.0), the accuracy ceases to increase.
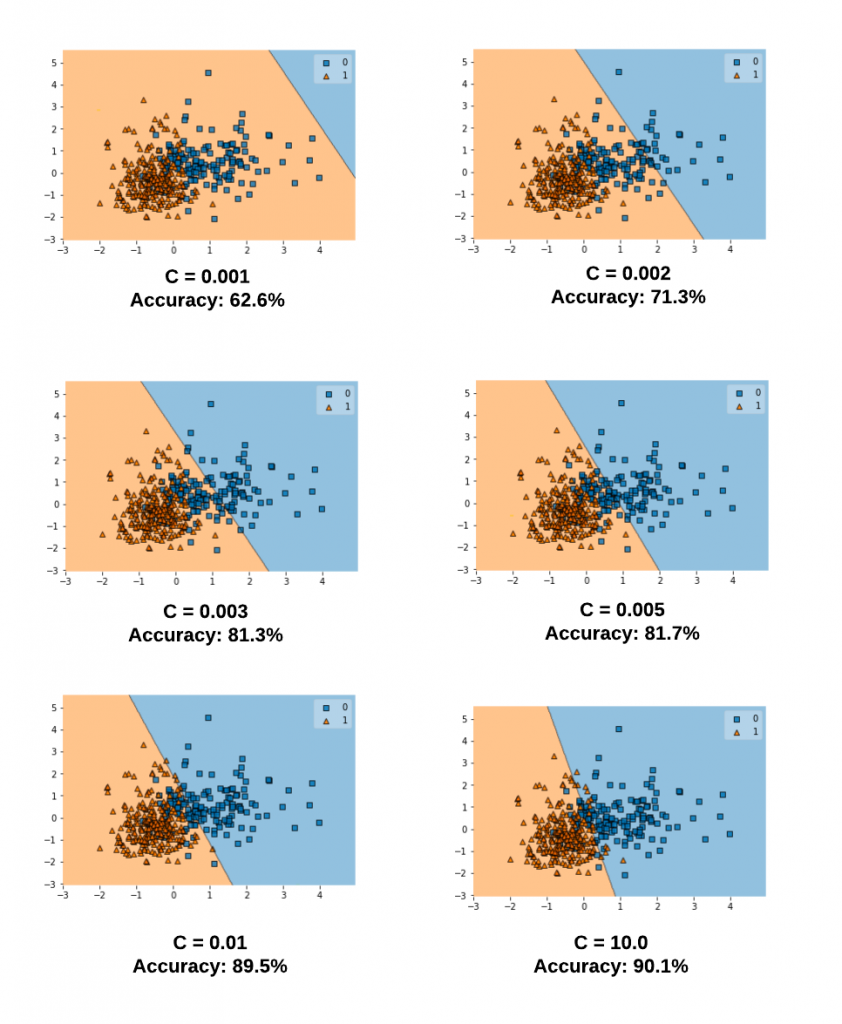
Let's take a look at different values of C and the related decision boundaries when the SVM model gets trained using RBF kernel (kernel = "rbf"). The diagram below represents the model trained with the following code for different values of C. Note the value of gamma is set to 0.1 and the kernel = 'rbf'.
xxxxxxxxxx
svm = SVC(kernel='rbf', random_state=1, gamma=0.1, C=0.02)
svm.fit(X_train_std, y_train)
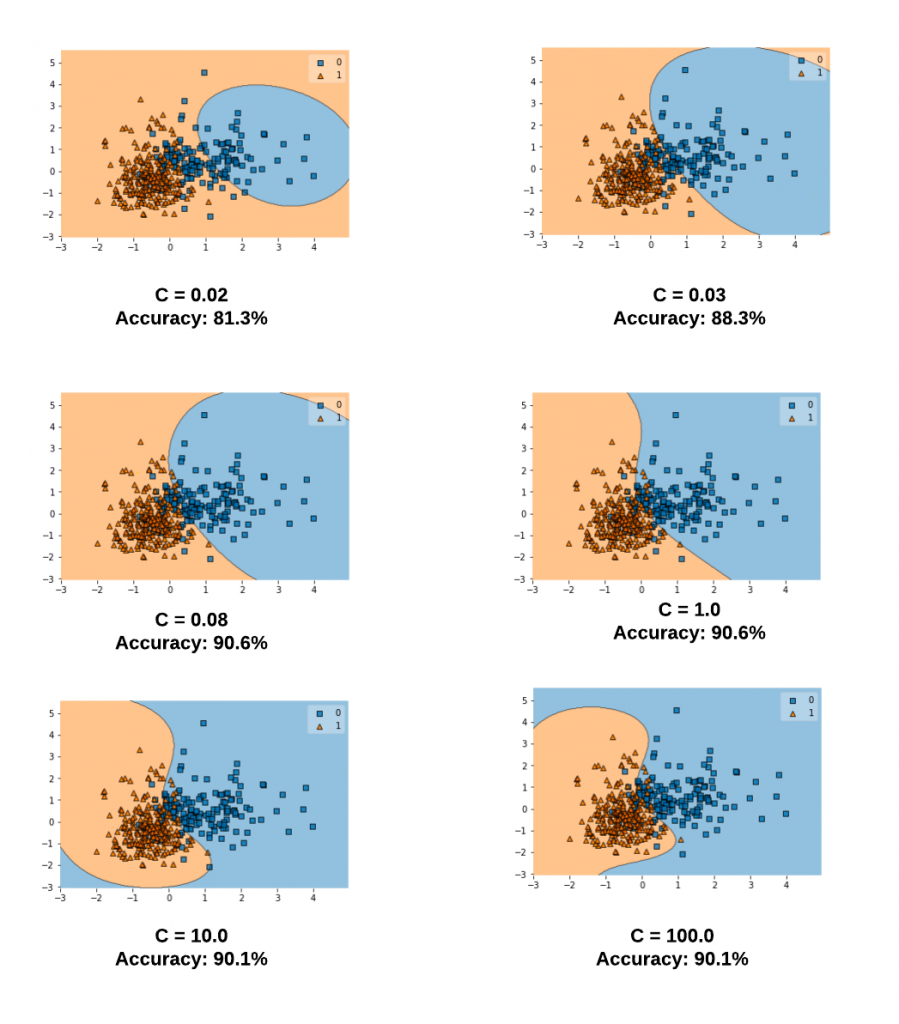
References
Here are some other posts on similar topics:
Conclusion
Here are some of the key points that is covered in this post.
- Gamma and C values are key hyperparameters that can be used to train the most optimal SVM model using RBF kernel.
- The gamma parameter defines how far the influence of a single training example reaches, with low values meaning 'far' and high values meaning 'close'
- Higher value of gamma will mean that radius of influence is limited to only support vectors. This would essentially mean that the model tries and overfit. The model accuracy lowers with the increasing value of gamma.
- The lower value of gamma will mean that the data points have very high radius of influence. This would also result in model having lower accuracy.
- It is the intermediate value of gamma which results in a model with optimal accuracy.
- The C parameter determines how tolerant is the model towards misclassification.
- Higher value of C will result in model which has very high accuracy but which may fail to generalize.
- The lower value of C will result in a model with very low accuracy.
Published at DZone with permission of Ajitesh Kumar, DZone MVB. See the original article here.
Opinions expressed by DZone contributors are their own.
Comments