How Data Observability Helps Data Catalog Initiatives
Here’s why some of the best data teams are investing in data observability before kicking off a data catalog initiative.
Join the DZone community and get the full member experience.
Join For FreeAs companies increasingly rely on data to drive decision-making and power digital products, it’s critical that this data is accessible, discoverable, and reliable.
For decades, organizations have relied on data catalogs to drive these initiatives. But are we missing the boat?
Don’t get me wrong: data catalogs are great, and we need them – they facilitate tighter governance processes and accelerate data adoption…but they can also become shelf-ware if you’re not careful.
One e-commerce organization shared an all too familiar story with me. They started their catalog initiative with passion and excitement, but it ended up being a heavy lift, and most data team members rarely used it.
The challenge was partly that data catalogs are often part of a larger governance overhaul that lengthens the time to value. Still, mostly, it was that the value wasn’t immediately evident.
Their users had a catalog of data assets with some context that was updated automatically, but they couldn’t differentiate the quality levels between datasets to determine what could be trusted.
Adoption was slow, which for data catalogs can be a time-to-value death spiral. Slow adoption means low ROI. Low ROI means limited business value for your data governance initiatives.
The most successful strategy when it comes to data catalog adoption? Make sure you can trust the data first.
Here’s why some of the best data teams are investing in data observability before kicking off a data catalog initiative.
You Get One Shot at Data Trust – Don’t Blow It
Data catalogs can boost data adoption and democratization, but as they say, “you only get one chance to make a first impression.”
In our experience, most business stakeholders will trust the data until they have a reason not to trust it. Unfortunately, it only takes a few instances—or a single incident, such as erroneous financial reporting for a public company—of conflicting or missing data to lose that benefit of the doubt. For example, the initial challenges Daniel Rimon, Head of Data Engineering at Resident, solved with data observability.
“Stakeholders and executives couldn’t access the most up-to-date data they needed to make decisions. It also negatively impacted relationships between business units. For example, if you’re in data engineering, it can strain relationships with a BI or analytics team…sometimes the CEO of the company would Slack me and my boss and me, ‘What’s going on? Didn’t we have any sales?’ So that’s my nightmare—getting a message that “the data is off. The data is broken,” said Daniel.
At that point, the relationship shifts, as does the burden of managing data quality. Executives and product managers turn to their domain analysts, who now have the final say on whether that data or report can be trusted.
This makes evaluating data quality a qualitative judgment based on an analyst’s gut rather than a quantitative exercise focused on service level indicators and agreements (SLIs and SLAs). It also defeats the original purpose of your data adoption initiative: leveraging self-service mechanisms to make the organization more nimble and data-driven.
On the other hand, you can take your data adoption journey to the next level by making data observability your first stop. Not only will data trust act as an adoption accelerant, but each dataset cataloged and discovered can be labeled and certified, conveying the appropriate level of support and trust it should engender.
One of my colleagues served as the Senior Vice President of Data and Insights at the New York Times and had to sign his name next to the data going to Wall Street every quarter. I bet his hand felt much steadier once he had a data observability solution in place and was on a path to certified “golden” datasets!
Data Observability Is a Quick Win for Your Data Team
The other side of that coin is data leaders can quickly earn a longer leash following a few quick wins, of which data observability undoubtedly qualifies. The beauty of machine learning monitors is configuration time is measured in minutes, not hours or weeks.
Time to value is quick. Fully automated, field-level lineage populates within 24-48 hours of integration with automated alerts for volume, distribution, schema, and freshness firing after a training period of about 2 weeks.
This was also the experience of Rick Saporta, Head of Data Strategy and Insights, at Farmer’s Dog.
“I wasn’t even expecting notifications yet,” Rick said. “I thought there would be another phase of work where we would have to set them up. I thought, ‘Okay, we got it configured, I’ll find some free time next week, and I’ll actually start setting it up so we’ll get these notifications.’
And then boom, I just get one in my inbox. Since then, we’ve gotten notifications for all types of different anomalies that I would not have thought to check. I keep thinking back at our original ‘6-month plan’ and how so many of the alerts we have since gotten from [our data observability platform] weren’t even in our original plan.”
While you can scale your sophistication through granular routing, detailed triage playbooks, and well-measured SLAs, there is no need to kick off with steering committees, governance tiger teams, or another slower, overarching organizational approaches requiring extensive codification or consensus.
Even better, the triage and data quality improvement process creates many of the connections, shared vocabulary, and intra-department processes that can be helpful during a 12-month data catalog initiative. You’re organically creating process by doing, which is what Vimeo found when they implemented data observability.
“We started building these relationships where I know who’s the team driving the data set,” said Lior, (now former) VP of data at Vimeo. “I can set up these Slack channels where the alerts go and make sure the stakeholders are also on that channel, and the publishers are on that channel, and we have a whole kumbaya to understand if a problem should be investigated.
You build a relationship with more data-driven teams. Some of them get excited about solving these things…It starts the conversation at the point where you’re setting up the expectations and escalates with your stakeholders, and [data observability] helps facilitate those discussions.”
Data Observability Allows You to Free Up Resources for Catalog Implementation
I’m truly amazed by what data teams can achieve when they no longer spend half their day fixing broken data. And it turns out; that it really is about half of their day.
Our recent State of Data Quality survey with Wakefield Research found that the average data professional in 2022 spent 40% of their day on data quality issues.
Wouldn’t it be nice to regain that extra 40% capacity and put it toward your ambitious data catalog and governance initiatives when you need all hands on deck? Not to mention, it is generally much easier to obtain additional resources once you’ve instilled data trust, as Lior also noted in the Vimeo case study:
“It would be hard to advocate for hiring more and taking on more risk for the business without creating that sense of trust in data. We have spent a lot of the last year on creating data SLAs or SLOs making sure teams have a clear expectation of the business and what’s the time to respond to any data outage.”
Data Observability Helps Optimize and Prioritize Your Assets for Cataloging
Data comes in fast and messy, so the vision of a fully cataloged data ecosystem is as tempting as a siren’s call. Your ability to pipe data is virtually limitless, but you are constrained by the capacity of humans to make it sustainably meaningful.
Virtually every grizzled data veteran has a horror story of how they failed, C-suite driven initiative to try and catalog every data asset run aground.
And while catalogs are a bit nimbler now, taking an absolutist/waterfall view to these initiatives is still risky. Data teams need to focus and move in an agile fashion.
Data observability solutions provide insights into the health, usage, and lineage of legacy data assets that can be deprecated. Real-time visualizations of the dependencies within your ecosystem can give you the confidence to deprecate a data set knowing that it isn’t part of an intricate chain that ends with your executive’s favorite dashboard. That keeps your environment more manageable and saves on computing, a win-win!
Data observability solutions can also leverage machine learning to understand how your data AND your organization are related to one another to make smart decisions on key assets. Those key assets make for great places to start your agile data catalog initiative.
Choose the Right Tool for the Right Use Case
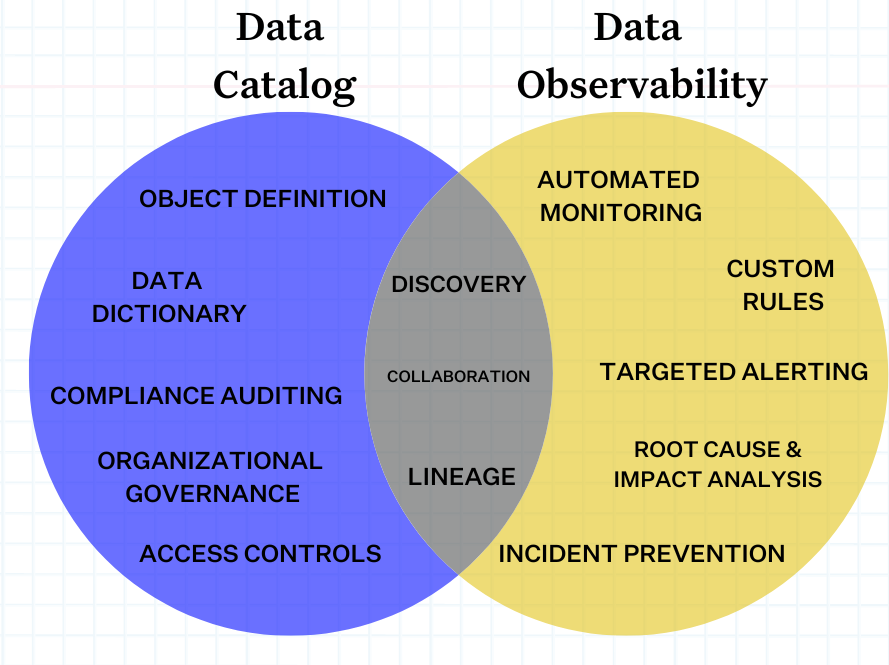
They are different solutions that work well together to tackle different use cases. As Datanami points out, data catalogs provide a lot of value from their ability “to provide a bridge between how business talks about data and how that data is technically stored. Nearly every data catalog in the market–and there are close to 100 of them now–can do that.”
Even when you consider shared features such as data lineage, the emphasis is different. Monte Carlo builds our data lineage to prevent data incidents and accelerate their resolution. The context we provide and our UI is designed for data engineers and other data-savvy users to accomplish this task as compared to something designed for a steward to help their cataloging activities.
Ultimately, the process of adopting new solutions always boils down to making sure you have the right tool for the right job. If you have the use cases above and need to prove value sooner rather than later, data observability might be your safer bet.
Published at DZone with permission of Lior Gavish. See the original article here.
Opinions expressed by DZone contributors are their own.
Comments