Data Processing vs. Process Management vs. AI?
Modern process management can help to build the bridge between data processing and Large Language Models (LLMs) in a data-driven business landscape.
Join the DZone community and get the full member experience.
Join For FreeIn today’s data-driven business landscape, managing data effectively is crucial for achieving organizational goals. While data processing systems play a vital role in collecting and organizing data, it is often overlooked that data should not be managed solely for its own sake. The true value lies in understanding how data can be leveraged to achieve business objectives, which is where the concept of process management comes into play.
Today we live in a world where data is everywhere and every enterprise knows about the value of data. But how do we handle data? How do we create, collect, and analyze it? This is the area of data processing software. We all use databases, spreadsheets, and data management systems to manage all kinds of data. Many software systems are built around specific data domains and we use this software to create and maintain the data.
In this context, however, it is often overlooked that data should not be managed for its own sake. The reason why we need data in companies is that we want to achieve certain business goals. And in this context, we come to the question of how corporate goals can be achieved at all. This is the area of process management.
So what’s the difference between data processing and process management?
The Problem of Data Processing
In a data processing system, we collect data according to a predetermined schema. This can be a database table, a spreadsheet, or any complex data structure, more or less. It is usually disadvantageous to change the data schema in such software systems afterward because each change either needs some kind of data migration or increases the complexity of the data structure. As a result, data processing systems often increase in complexity over time as more and more disparate data has to fit into a general schema. This can lead to software systems becoming unmanageable or development costs rising to astronomical heights over time. Microservice architecture is a common approach to stop this development and consistently create independent smaller data silos from the start. But also in a microservice architecture, the focus still often lies on the data.
The Idea of Process Management
On the other hand, we have the concept of process management. In this area, we are focusing on the question of how to process data and not how to put data in a rigid schema. The idea of process management software is to process data in a highly flexible way. The main question the process management tries to answer is: who needs what kind of information in a business process?
These processes are highly versatile because our business requirements are changing much faster in today’s rapidly evolving business landscape. Thus, process management also focuses on the question of how data can be processed quickly and flexibly if our business landscape evolves.
BPMN 2.0
The business process management notation (BPMN) is the industry standard to describe and execute business processes. It enables enterprises to describe how data should be processed, who is responsible, and how certain situations should be handled. Besides a workflow, a BPMN model may include the description of responsibilities as well as various business rules.
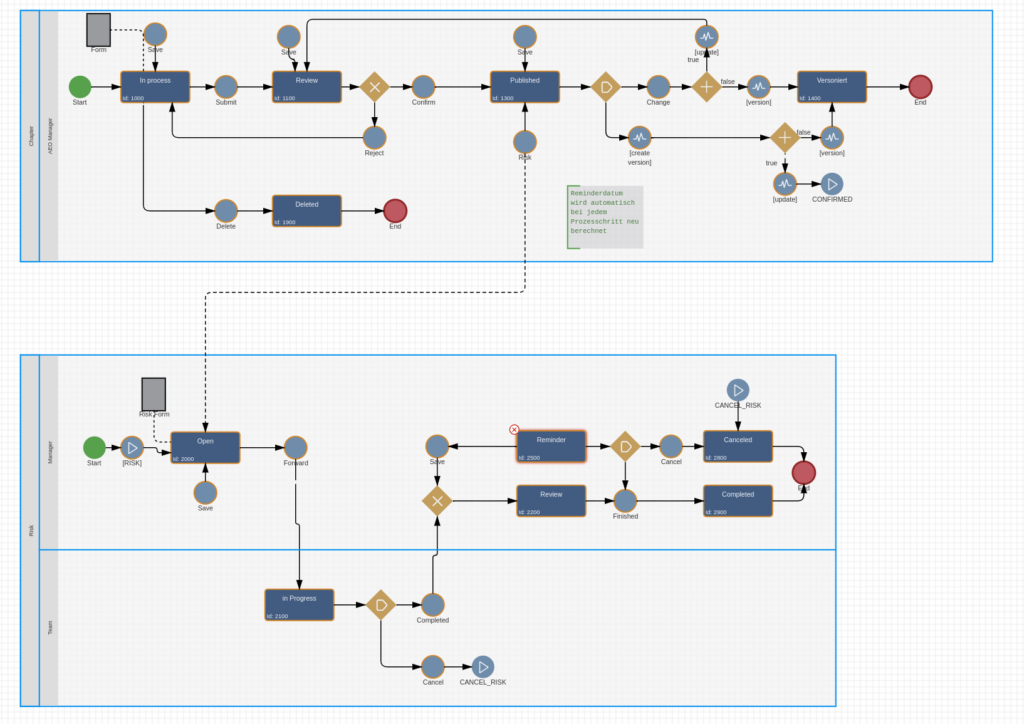
One major advantage of BPMN 2.0 is that software systems can execute those process models automatically. There are a lot of open-source BPMN engines in the market that can process and execute this kind of workflow model. A BPMN process model can be created with open-source tools like Open-BPMN easily within minutes. Changing the process flow can also be modeled in a live system, giving an enterprise the flexibility to manged its own processes much faster.
Process Management and AI
In general, process management solutions handle data in a more flexible, document-centric way. A business process is typically not bound to a specific data domain. Think, for example, of a quotation process that leads to an order process and finally ends in an invoicing process. The data domain changes constantly over the life cycle of the process. This flexibility of process management simplifies data management on a higher level. Typically, you don’t ask what kind of data was processed, but look for answers to questions like:
- Why did we need to process this kind of data?
- Who knows about specific data in our company?
- When did certain data events occur?
A process management solution collects all this information and Artificial intelligence (AI) plays an increasingly important role here. Large language models (LLMs) are optimized to handle this type of unstructured data and can quickly find answers to these types of questions. One approach to combining the flexibility of BPMN with the power of AI is to enable a process model to create prompts in the context of a specific business process. Prompts can be embedded into a BPMN model. This empowers companies to combine new questions within the processing flow of their data.
Conclusion
Process management offers a flexible and adaptable approach to data handling, focusing on how data can be processed to support evolving business requirements. By combining the industry-standard BPMN 2.0 with the power of artificial intelligence (AI), enterprises can gain a deeper understanding of their data and make informed decisions that drive business success. Modern open-source solutions enable organizations to create prompts within specific business processes and leverage AI capabilities to extract valuable insights from unstructured data. As the business landscape continues to evolve rapidly, process management's ability to adapt and harness the potential of AI will be critical to maintaining a competitive advantage.
Published at DZone with permission of Ralph Soika. See the original article here.
Opinions expressed by DZone contributors are their own.
Comments