Where the Data Silos Are
You’ve heard of shadow IT, but what about shadow data? Find out where your data silos are—and how to get rid of them.
Join the DZone community and get the full member experience.
Join For FreeSomething interesting happened the other day. Our company experienced a minor data incident that our data observability platform didn’t catch for the simple reason that the data was trapped in a silo.
This got me thinking about other places data hides, not just from data observability platforms but from the scrutiny of the data team itself — and how these risks can impact our ability to successfully implement more modern or even experimental programs like data mesh. We sometimes refer to these as data silos, but really it’s the data equivalent of shadow IT.
Shadow data (or data silos) arise when:
- Autonomy and speed are prioritized over technical standards;
- Data access or resources are limited, forcing teams to work around existing systems and processes; or
- Data consumers decide to deploy their own point solutions rather than work with you.
But instead of waving the white flag, consumers often find a way to get what they need outside of the watchful eye of the data team — and that’s risky.
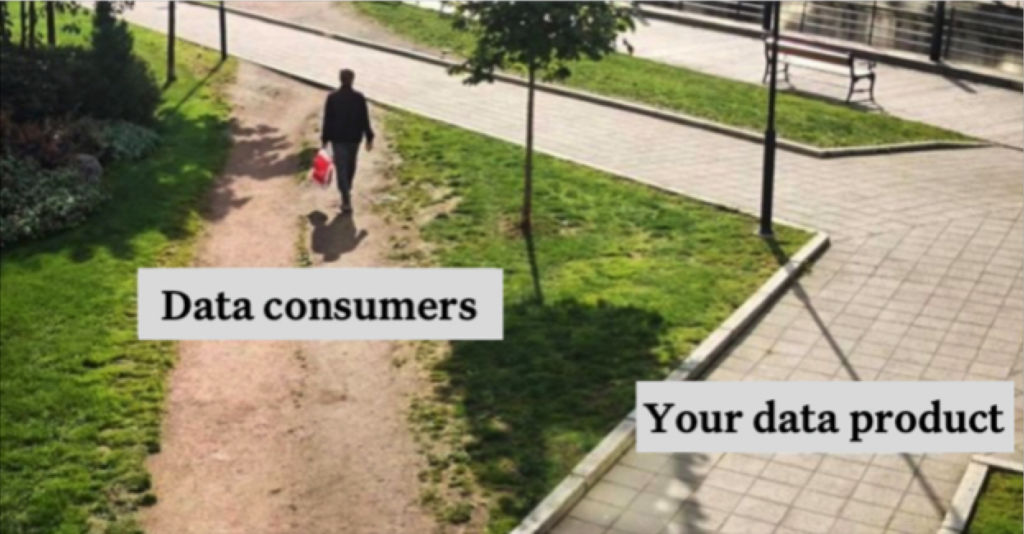
Data silos present numerous risks to data consumers and the data team alike, such as:
- Fragility: I’d wager John on the finance analytics team doesn’t have systems like dependency management and anomaly detection in place for his data set. And when executives come to you to ask why this metric is wrong, it’s going to be hard to debug when you don’t have any idea how it’s produced. Either way, this creates a risk that valuable business data may be lost.
- Knowledge loss: If a data “power user” leaves, you don’t want to have to figure out how to reverse engineer the esoteric system you have now inherited. Not only will this be a poor allocation of resources, but their system may create untold headaches before it’s discovered as an issue (one company saw millions in revenue impacted when one of their predictive algorithms was discovered to be running on autopilot following the departure of a team member).
- Security: “I didn’t know about it!” won’t prevent regulators from handing down a fine for improper handling of personal data. For GDPR, that fine can be up to 4% of the company’s revenue–ouch.
- Spotty Access: Analysts get grumpy, and rightly so when they can’t get access to what they need because it can’t be joined to their canonical user tables. These data silos create either opacity or the need to duplicate processes — neither of which is a positive outcome.
- Blinkered decisions: While data consumers can move quickly with user-friendly point solutions, for complex decisions, they may need an expert to think through experiment design, sampling bias, and confounding factors. An entire department could be circling the wagons and marching towards a goal that won’t fundamentally add value to the business or that’s being measured in an arbitrary fashion.
So how can data teams identify the data silos that lurk in the shadows? Can they use it as an opportunity to spot weaknesses within their data platform and bring consumers into the fold, either by encouragement or force?
In my opinion, the answer is yes. Let’s take a look at where the data silos are and how to break them.
Data Silo 1: Transformations and Pre-Aggregations
But before we talk about data consumers, let’s not let ourselves as data professionals completely off the hook. We create our fair share of data silos in our quixotic attempt to meet the ever-increasing demand for data while working within the realities of our team’s capacity.
The data incident we experienced the other day was a result of adding DataDog to our tech stack, which introduced some tracing information into the data structures flowing into our application. And yes, believe me, I’m aware of the irony here.
When that data persisted into S3 and then ingested into Databricks, it was only partially loaded, so our pipelines had missing data.
Our post-mortem concluded that our Spark jobs were overly complex with too many transformations. What we should have done as a best practice is to break the job up into a series of smaller transformations at key checkpoints that wrote to tables that could be monitored, which would have triggered a Monte Carlo volume alert.
I’ve seen a similar scenario when dealing with old-school ETL and business intelligence implementations. Data was pre-aggregated for performance, and the underlying layers were invisible to an analyst responsible for diagnosing surreptitious declines in key metrics. The analyst was lucky if they knew how the underlying data was transformed, let alone able to successfully find the cause of the decline.
Some of this behavior shifted with the move from ETL to ELT. Now, these days, you are more likely to see data teams establish best practices around breaking up transformations into clear steps (e.g., events => sessions => users => campaigns) rather than writing three pages of SQL that only the owner can decipher.
While business intelligence tools will tout their data prep capabilities, this can wind up turning into another silo of business logic unavailable to teams outside of the tool. Clearly, there are some benefits to last-mile manipulation, and maybe rules like “no more than SELECT*” within BI are too draconian, but any reusable semantics must be widely available to your team.
Solution: Break up complex transformations or SQL queries into distinct checkpoints that write out to tables that are monitored for data quality. Ensure your business logic isn’t locked into a single tool only accessible to part of your user base.
Data Silo 2: Spreadsheets
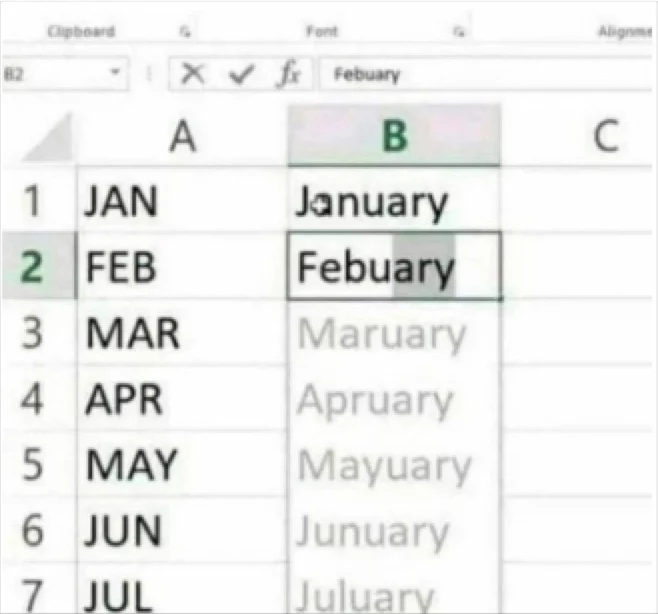
Spreadsheets remain the most successful way to democratize data in many organizations, even if they may be an occasional source of pain and derision for data teams.
Our partners in the finance department can do remarkable things with a PC and a VLOOKUP, and in my experience, there are few better ways to democratize the prototyping of new data than by dropping a table into Google Sheets and collaborating with partners to manually append tags that are meaningful attributes for them to see in analysis.
A spreadsheet becomes a data silo when it moves from prototype to production. Or in other words, if you see the same spreadsheet playing a role in a business operation more than once, it’s time to move the logic upstream and create something more systemic.
Solution: A hack for data teams is to periodically review the mammoth spreadsheets that are being used to guide the business or maybe sitting in a shared drive, spreadsheets that likely have some gnarly formulas or macros sitting on top of data that are supplied by your team according to their specifications.
This review will often reveal ways to address gaps in your data platform and can help you bring transformations upstream to increase observability and scalability. You may even unlock new opportunities to standardize metrics such as lifetime value (LTV) for wider applications and produce them at a far more granular level.
Data Silo 3: The “All-in-One” Solution – ESP, CDP, DMP, A/B
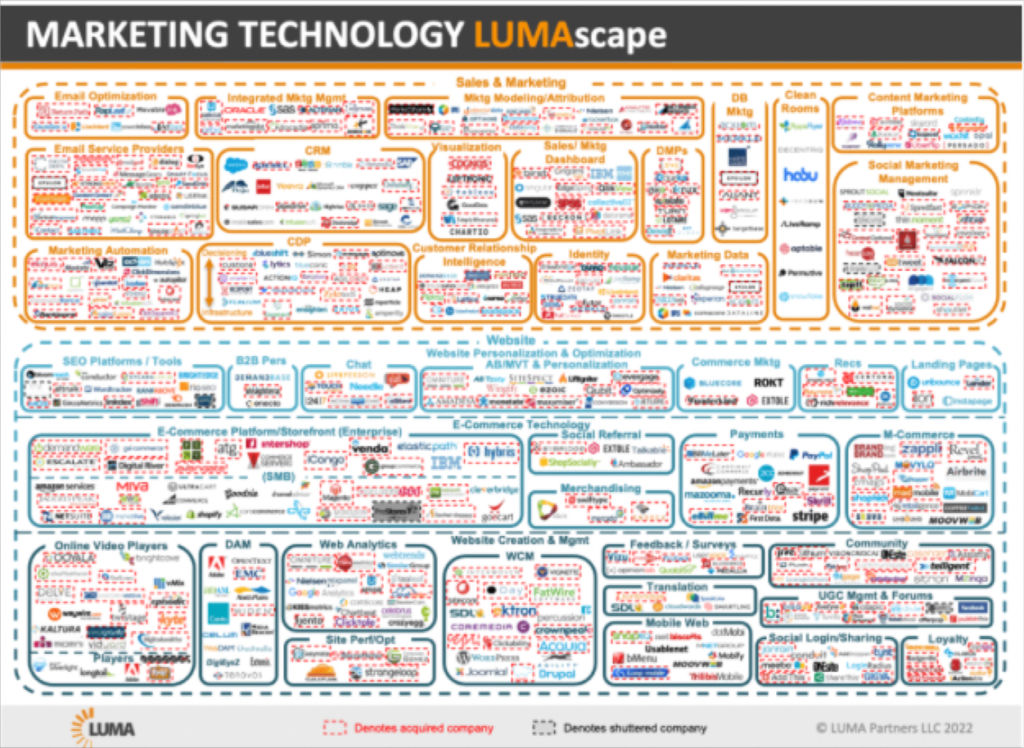
When done wrong, these can be the hardest silos to reign in.
Much of the marketing tech stack, like email service providers and marketing automation platforms, rose to prominence ahead of the advances in modern data warehouses. This meant the most expedient way to drive impact was with an all-in-one solution that collected, managed, and served data directly to the marketer. Admittedly, I was once selling the dream of a “single line of JavaScript” for running experiments that allows you to avoid any further conversations with “IT.”
Some of the most pressing problems created by this silo are that these systems quickly experience an ungoverned sprawl of customer segments (product page repeat visitors Oct 2020), and the segmented campaigns often suffer from a lack of measurement.
Back in my consulting days, I assessed that one large telco was “micro-targeting” less than 1% of their customer base because they were unknowingly filtering campaigns down to customers that satisfied every attribute within their complex segmentation.
But we turned a corner a few years ago — data teams and technologies are now capable of being fast and flexible enough to operate at the speed of business while also unlocking new opportunities with the richness of data in the warehouse (“hey, want to optimize for LTV instead of click-through?”). Marketers and other business partners see the value in going through, rather than around, the data team.
These marketer-first solutions are adapting. Whether you opt for a modern CDP or reverse ETL to transport data into the hands of marketers, the must-have feature is to have a collection, transformation, and basic segmentation operating on your enterprise data warehouse.
Solution: I’ve found the best way to get marketing teams onboard is to create systems that respect and address their need for speed and autonomy while partnering to ensure strong governance and measurement are part of the solution.
My Recommendation? Proactively Remove Silos
It can be tempting to let sleeping dogs lie, but, in my opinion, data teams should proactively work to remove data silos or any “shadow data” systems.
I’ve typically opted for the Field of Dreams approach — “if you build it, they will come.” (The approach is probably more akin to gathering requirements, scoping the project, getting approvals, building a minimum viable product, getting feedback, iterate and they will come — but that is not nearly as pithy).
But if you build it and they don’t come, then you need to address whether that’s a failure of your technology solution, a lack of organizational buy-in, or something else altogether. Then you need to find a solution that puts you on the right path to breaking down silos.
After all, data is ultimately the data team’s responsibility, and consumers will do what they need to do to access it. Our best path forward as data leaders is to accept this reality and take steps to mitigate it.
Published at DZone with permission of Shane Murray. See the original article here.
Opinions expressed by DZone contributors are their own.
Comments